Department News
[Hankyung Business] Prof. Byeng Dong Youn “Predicting Faults 6 Months Beforehand… KEPCO is Our Biggest Client”
Author
관리자
Date
2021-01-28
Views
1074
[HELLO AI=12 AI Frontiers interviewed by Kyoung-jun Lee - Prof. Byeng Dong Youn, CEO of OnePredict]
- Started in a university lab in 2016, annual growth at 40% … “Without accumulated expertise, AI is useless”
[Hankyung Business=by Hyeonju Lee] There is a company that predicts faults of industrial facilities with AI. OnePredict offers prognosis-based management solutions, combining physical knowledge industrial facilities and AI technology. Professor Byeng Dong Youn of SNU Dept. of Mechanical Engineering, hailed as the best expert prognostics and management, founded OnePredict in October 2016 with four of his students as a lab start-up. Last November, they moved headquarters from SNU to Teheran-ro, Gangnam, further expanding their business.
- Started in a university lab in 2016, annual growth at 40% … “Without accumulated expertise, AI is useless”
[Hankyung Business=by Hyeonju Lee] There is a company that predicts faults of industrial facilities with AI. OnePredict offers prognosis-based management solutions, combining physical knowledge industrial facilities and AI technology. Professor Byeng Dong Youn of SNU Dept. of Mechanical Engineering, hailed as the best expert prognostics and management, founded OnePredict in October 2016 with four of his students as a lab start-up. Last November, they moved headquarters from SNU to Teheran-ro, Gangnam, further expanding their business.
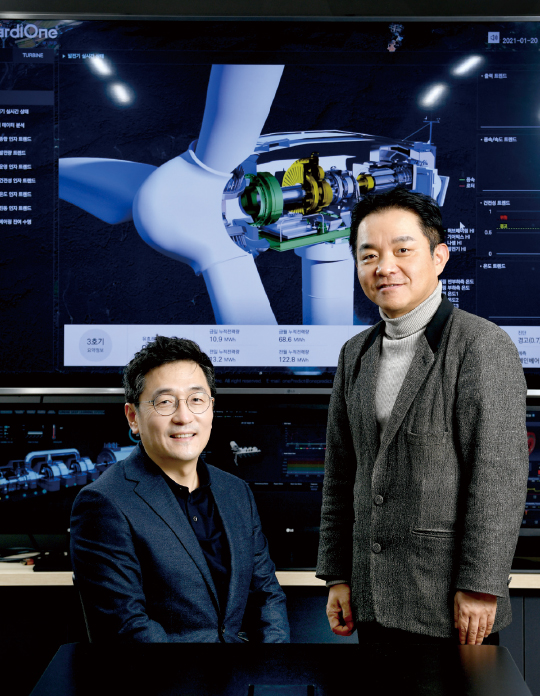
Prof. Byeong Dong Youn (left) and Kyoung-jun Lee / by Kinam Kim
Last-stage blades (LSB) are low pressure turbines of a power plant. They are a key component responsible for about 10% of total output of a power station. With the increasing output of power station, the length of LSB has also been growing longer, causing the risk of major accidents such as power plant stoppage or fire. When the LSB malfunctions, notly does the power station shut down, it may also lead to other facilities to break down. Until now, it was possible to analyze the causes of LSB breakdowns of a 1000MW turbine, but performing prognostics was very limited. OnePredict introduced world’s first prognostics system that analyzes vibration data from 1000MW blades via deep learning. This comprehensive technology that identifies the location, cause, and follow-up actions of faults was selected ase of ’10 Technologies of the Year’ in 2019 by the Korea Association of Machinery Industry.
OnePredict’s ‘GuardiOne Wind,’ which monitors facilities in real-time, can predict faults in major parts 3 to 6 months beforehand. The Korean wind power market requires around 4 months to fix the apparatus when it malfunctions. For instance, when the main bearing breaks down, the entire system stops for a maximum of 150 days from bidding to installing a newe. OnePredict analyzes vibration data with AI, and performs prognosis major parts such as the main bearing, the gearbox, and the generator with GuardiOne. It successfully predicted faults of a gearbox of a unit in Western Power Co.’s wind farm 4 months beforehand, resulting in a return investment of 520 million won.
These are the main cases where OnePredict’s GuardiOne was used to solve problems. GuardiOne is a software used in a variety of industrial fields that examines facilities and performs prognostics faults and expected life. It consists of four types according to its usage: GuardiOne Turbine, GuardiOne Transformer, GuardiOne Bearing, and GuardiOne Wind.
Digital transformation is happening worldwide. In industrial fields, there is an increasing need for predictions using industrial data. For instance, January 8th, a fire broke out at a facility in Shin-Bupyeong substation, causing power outage and water stoppage in 38 thousand households. Industrial prognostics offers a way to prevent financial loss due to large-scale accidents and power generation stoppage, as well as protecting residents from abrupt inconveniences. OnePredict mainly uses GuardiOne for power plants, transmission stations, and substations. It also deals with other industrial areas such as smart factories and mobility.
OnePredict defines Industrial AI as a combination of physics-related domain knowledge and AI. It offers prognostic solutions in software form by combining expert knowledge industrial facilities with AI. It is also unique in its beginnings as a university laboratory startup, accumulating fundamental knowledge in the university to apply it in the field. For the first time in Korea, it has received a total of 9 awards in Global Data Challenge hosted by PHM Society, proving their technological prowess.
Prof. Byeng Dong Youn, CEO of OnePredict, plans to invest more resources in 2021, moving up the J-curve. Since the foundation in 2016, the company has seen an average annual sales growth of more than 40%, and plans competing more fiercely in the market. It also will increase in size, expanding from 53 employees to 80 employees.
“We are a company that makes ‘Industrial Digital Brain’ with industrial AI technology. OnePredict’s greatest added value is created via digitalization,” said Prof. Youn.
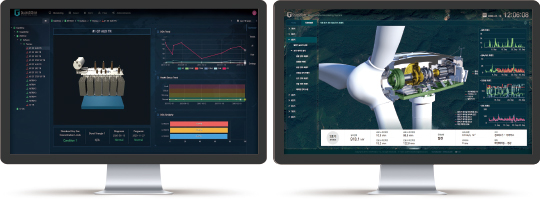
OnePredict’s ‘GuardiOne Transformer(left)’와 ‘GuardiOne Wind(wind power solution)’
Prof. Kyoung-jun Lee (hereinafter Lee) : “Is OnePredict a consulting firm, service company, solution company, or a platform company?”
Prof. Byeng Dong Youn (hereinafter Youn) : “We are a platform-oriented company. But platforms are not created overnight. So currently we’re offering both solutions and services—developing solutions and expanding into service business. Since last year we’ve been reducing our reliance outsourcing services and increasing our services as a subscription model.”
Lee : “So it’s a pay-per-transformer type of model.”
Youn : “Each transformer gets charged. The cost differs according to the transformer’s capacity. Our biggest client is KEPCO, as well as public institutions such as Korea Midland Power Co. and Western Power Co., and other private firms. For example, petrochemical plants and large-scale plant facilities require hundreds to thousands of transformers.”
Lee : “If they have transformers, do they also have their own power plants?”
Youn : “No, but they demand lots of electric power. When a power station supplies power, first it goes through transmission then gets decompressed in the distribution stage before it can be used as industrial electricity. That’s why industrial facilities such as petrochemical steelmaking plants have transformers. Our solution is used in the beginning and the very end of the power generation process.”
Lee : “The government has to change as well. It’s supposed to be a service model, not outsourcing.”
Youn : “The biggest problem is that unlike hardware, software lacks a clear standard in determining appropriate prices, so there’s a gap between what we think and what the user thinks. I believee way to work around this is using the service model. The support of governmental policies is crucial, but I think convincing the users is a more important issue. People need to accept software as a service model, or think of it as a product you pay for, like hardware.”
Lee : “Is the transformer solution taking the lead as a profit model?”
Youn : “Yes. We are supplying ‘GuardiOne Transformer’ from a subscription point of view. Products like ‘GuardiOne Turbine’ go to public institutions. All that goes into the public sector is commissioned. We decide a supply price then offer our products. Recently we’ve been talking about how to offer this as a service to private firms.”
Lee : “The transformer itself is not a connected product. What kind of hardware is installed with the connected apparatus? Or is there already some type of hardware control?”
Youn : “Sometimes they have the hardware. In terms of transformers, sometimes we install the hardware itself or bring the hardware everyce in a while to take measurements. There are maintenance companies like that. For example, they would get the data from the transformerce a year. We offer our services based that data. Recently, we’ve also been taking measurementsline, though it’s a small market as of now. But we expect it to grow and are preparing our software supply accordingly.”
Lee : “So transformers’ data don’t have to be collected more thance a year.”
Youn : “Once a year in general, if there aren’t any issues. The period is shortened when we find out a problem. Our product can make predictions that are 6 months, or a year ahead. If any issues come up in the prognosis, we take measurements in a 6 month or a 3 month period to examine things better. These measures are also included in our service.”
Lee : “What are some of the main successes you’ve had since the foundation of OnePredict?”
Youn : “We’ve used ‘GuardiOne Wind,’ a wind power solution, for the Korea Western Power Co.. Wind power needs things like main bearings, gearboxes, and generators, which are not ready-made. They are all made to order. If there were ready-made products, we’re able to stock up first then replace the parts when they break down. However, for example, if the main bearing malfunctions today, the power plant will call for bidding. It takes 4 months from placing the order, production, transportation, to installation. In the meantime, the power generator remains standstill, unable to generate electricity—losing opportunity costs. We can compensate for these potential losses that can happen during the 4 months of downtime. We’ve had four successful cases from the Korea Western Power Co., with high rates of return investment. Thanks to these successes, we’re taking private contracts instead of competing in open bidding.”
Lee : “How do you determine a successful case?”
Youn : “First we notified the power plant upon discovering issues, and they asked the manufacturer, who at the time said there wasn’t anything wrong. But actual malfunction occurred 4 months later. The issue was with the bearing in the 3rd gear of the gearbox. We had told them the same thing and it actually happened. We’ve also supplied our transformer solution to another client and detected faults. The following week, the person in charge at the site noticed the odd vibrations, allowing for a quick response. We were able to prevent a huge accident.”
Lee : “That’s very interesting. How come we don’t see more of it in the field while OnePredict does it?”
Youn : “That’s the motivation behind our technology and also what we offer to our clients. There are plenty of experts in the industrial site. They hear sounds and check vibrations, gathering information with their senses. Recently, with technological development, alarm goes off when the sensors notice the vibration or the temperature value going above a certain level. But this is all empirical, tacit knowledge. It’s very difficult to transfer to other people. We wondered if this kind of tacit knowledge could be formalized—and that’s where this research began. We first focused domain knowledge-based analyses, then used AI to examine the data from IoT. We developed our solution by combining the two.”
Lee : “So the knowledge of domain experts can become a mathematical model.”
Youn : “That depends. For instance, things like the rotary system can be modeled mathematically. However, if the rotary system has multiple axes and grows in scale, modeling becomes difficult. In that case we figure things out with physical knowledge such as vibrations.”
Lee : “So externally observable phenomena is a factor in judgment.”
Youn : “I watched a TV program called ‘Life Master’ when I started this research. There’s a person who examines the quality of urinals, checking for cracks by tapping three times with their rubber hammer. Noe else can tell, but they can, with their accumulated tacit knowledge. I wondered if this could be systemized, so we can pick up the sound with a microphone and analyze it with a mechanical method.”
Lee : “When did you start this research? I’ve looked up your papers, and you’ve been doing this for about 20 years, beginning from 2003.”
Youn : “I got my Ph.D. in 2001, so since then. The work I had been doing until then wasn’t so unrelated. I had conducted research reliability-based design. Prognosis can also be viewed from the point of reliability of facilities. Reliability approach is based some type of a model or physical foundation. The current research expands upon it, combining data-based approach.”
Lee : “There are predictions of domain expert or domain model becoming unnecessary, with AI and data-based approach. What percentage do the domain model and data-based model take up when you think about their contributions to OnePredict’s performance?”
Youn : “Half-half, perhaps. Depends the product. Regarding rotary systems like wind power, the domain model (physics model) would be 7 to 3 or 8 to 2. On the other hand, transformers don’t have a clear governing equation that we can use. In this case, data-based AI technology is used more than the physics-based model—perhaps 8 to 2 or 9 to 1.”
Lee : “Is domain knowledge the main starting point?”
Youn : “Domain knowledge is absolutely required. AI is important, but AI cannot offer all the answers. From my experience, we can’t build a good solution without domain knowledge. That won’t change after 100 years. Sedol Lee lost to AlphaGo, but he didn’t lose to deep learning, but to a combination of domain knowledge and deep learning. The domain knowledge of Go is incorporated into deep learning. We will always need domain knowledge.”
Lee : “AlphaGo Zero, revealed in 2017, was able to learn without studying records, which wasly possible because Go was a mathematical model. I’ve been wondering if domain knowledge is needed outside of AI, machine learning, deep learning, and enhanced learning, and you’ve given me a clear answer.”
Youn : “Of course, AI is necessary. Our domain knowledge is not that advanced, and AI fills in that gap. Also, we can keep improving the diagnosis model with AI. AI is crucial in this sense. That’s our difference compared to other companies such as GE and Siemens. They are also delving into AI these days, but we’ve been designing products from the start, keeping in mind how to combine two drastically different systems of knowledge. That’s what differentiates us from others.”
Lee : “When companies like OnePredict become common, wouldn’t the manufacturing firms come up with their own services?”
Youn : “Major companies have the capacity, finances, and their own site for testing. They have plenty of opportunities to do it their own. We don’tly focus major companies. In terms of smart factories, our main clients are small- and middle-sized businesses. As of now their willingness to pay for our solutions (willing point) isn’t that high, but as our technology improves and gains price competitiveness, I expect that will change.”
Lee : “Wouldn’t the transformer manufacturer be willing?”
Youn : “Of course they are, and in fact, they are doing it. But our strong point is that, unlike manufacturers, we have experience dealing with products manufactured by multiple different firms. Our technology and product can be broadly applied regardless of brands. Manufacturers may have lots of knowledge design, but their management technology and management data is relatively lacking. On the other hand, we’ve gathered data working directly with operators. Our product covers multiple brands.”
Lee : “Which field has the most needs for IoT?”
Youn : “Electricity, I believe. A number of IoT devices are implemented in the process of transmitting, transforming, and distributing power. Transformers used to require an actual person to take care of itce a year. Now, it’s allline. It’s embedded.”
Lee : “So OnePredict doesn’t actually make and deliver the product, butly deals with software.”
Youn : “Hardware is not our area. Except for certain rare cases where including hardware is beneficial for product constitution, it’s all software. For instance, industrial robots or industrial motors are better if they are a single product with IoT included, and that’s when we would design hardware.”
Lee : “What’s the biggest issue at hand?”
Youn : “Developing new products. We have our own internal process, but we’re trying to make things more efficient from product design to development. We’re working standardization. The first half of the year will be focused that project. After that, I expect that any product will go through the same process and template, from the stage of product design to development.”
Lee : “What sort of plans do you have for the global market?”
Youn : “OnePredict aims to become a global firm. But with the spread of COVID-19 last year, we werely able to sell products indirectly. We plan using webinars to break into international markets during the first half of the year.”
Lee : “What do your employees look like?”
Youn : “Currently we have 53 employees and are recruiting more. We plan bringing in more people in the software field. By the end of the year we hope to have around 80 people. Two-thirds of our employees work in research and development. Among those, 40% have a background in mechanical engineering, and 30% in computer engineering.”
Youn : “People with domain knowledge is learning AI and creating models.”
Youn : “We call them solution developers. With bases in mechanical, electrical, chemical, and industrial engineering, we learn AI to develop solutions. One thing I always say to our developers is to become ambidextrous. These days, solution developers need to learn more than just solutions. In the end, if they work to improve themselves and learn more skills for themselves, the company also benefits from it.”
Lee : “When did you start incorporating deep learning in research?”
Youn : “I started using AI in late 2000s, deep learning in early 2010s. At that time it was difficult to get government projects with deep learning, but things have changed since AlphaGo. Since then, there’s been a surge of government and industry projects.”
Lee : “Any room for policy or system improvements in government or the National Assembly?”
Youn : “Raising awareness for software. Indeed, there are certain policies that outline an appropriate price for private contracts if you have software certification. But going through that process requires a lot of administrative support. There has to be a separate administrative team for taking care of documents, gathering back data, and reapplying for certification. The process is very complex and expensive. We are willing to spend our finances if the results are guaranteed, but they are not, which is what makes us hesitate. Also, gaining access to public data isn’t so easy. In the period of 4th Industrial Revolution, data itself is raw material, but we don’t have access to this. We don’t get meaningful information. This is detrimental to our national competency. Public data must become more accessible for the future industrial competitiveness of the country.”
OnePredict as seen by Prof. Lee…
-AI company focusing prognosis
-Industrial domain knowledge and deep learning technology are combined in a solution for turbines, wind power, and rotary system-based machinery such as bearings and transformers; gradually turning into a subscription business model
-Accurate predictions of fault location and time period allows it to perform well in industrial areas with a long lead time,
-Introduced domain knowledge and machine learning in the traditional fault diagnosis field 20 years ago; rapidly growing with the AI boom
-An exemplary case of a professor-led startup
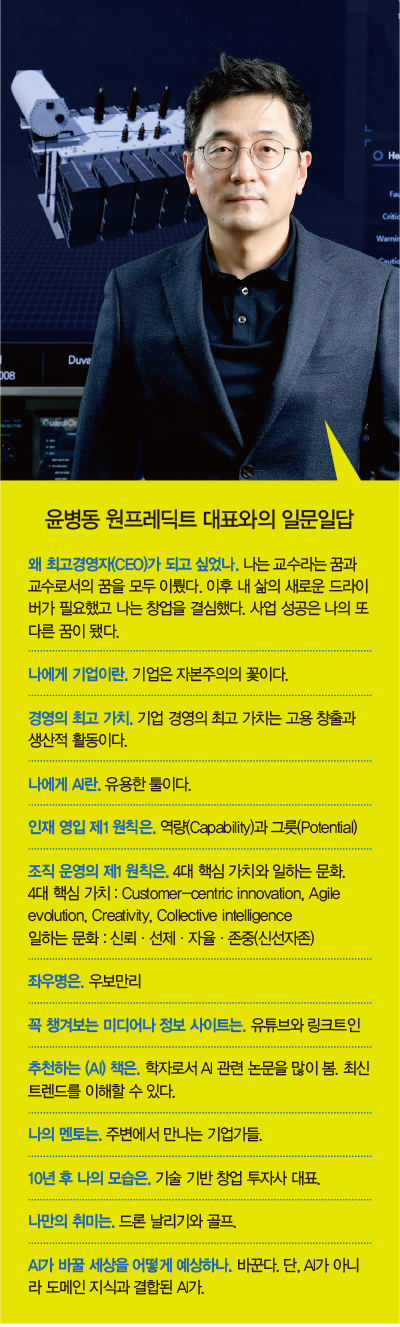
Original article : https://n.news.naver.com/article/050/0000056491